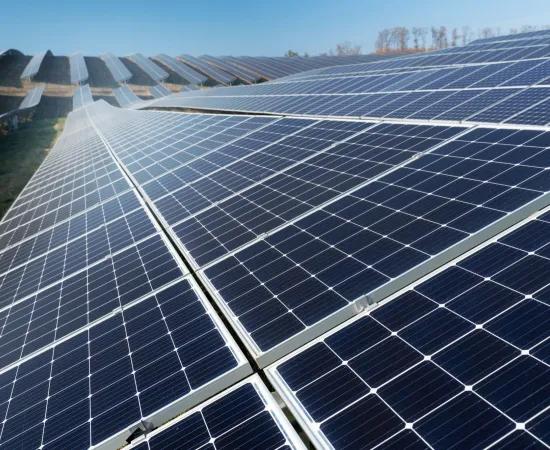
BitWind | Fleet-based Artificial Intelligence for Fault Detection and Maintenance Optimisation for Offshore Wind Farms (BitWind)
The aim of the project was the development of novel artificial intelligence techniques on data of operational offshore wind farms. The use case developed during the project focused on automatic performance degradation detection and prognosis of the expected lifetime of components.
Context
Wind power is one of the fastest growing renewable energy sources, and investments in wind energy are expected to grow considerably in the next decades. Giant wind turbines are an absolute necessity for tackling climate change, a topic which is high on the agenda of all EU countries.
In order to remain competitive with other sources of electricity generation, offshore wind energy needs to bring down the associated costs significantly. This not only includes the upfront investment costs related to the planning, financing, manufacturing and installation of offshore wind farms but also their operation and maintenance (O&M) costs (operational costs count for 25% of the costs of electricity from offshore wind).
BitWind aimed at developing novel artificial intelligence techniques for automatic performance degradation detection and prognosis of the expected lifetime of components. These innovations should lead to new services and a strategic cost reduction for the existing and new concessions in Belgium.
Objective and results
The goal of this project was to detect degrading trends in performance based on the detailed characterization of the dynamic behavior of the farm and its constituting turbines, which allows to determine normal degradation trends and cycles and identify abnormal trends, steps, spikes or noise levels.
Approach
To achieve the above-mentioned objective the partners:
- applied advanced multivariate time-series analysis methods (e.g., Gaussian processes) that allow to take into account both seasonal (daily, yearly) trends as well as the operational context (e.g., environmental conditions) in which the turbines are operating. Data from healthy turbines are used as additional pieces of information for benchmarking and training the dynamic models.
- detected underperformance by comparing the performance trend of a particular turbine across different axes, namely 1) with the performance trends of the assets in the same group, 2) with the assets in the same farm, 3) with assets in similar operating conditions (across farms), and 4) with assets of a similar type (i.e., same manufacturer, similar deployment time, similar maintenance schedule, etc.)
Funding
- Funding agencies: FOD Economie, K.M.O, Middenstand en Energie
- Modality: Energietransitiefond by FOD Economie, K.M.O., Middenstand en Energie